RECAST: Enabling User Recourse and Interpretability of Toxicity Detection Models with Interactive Visualization
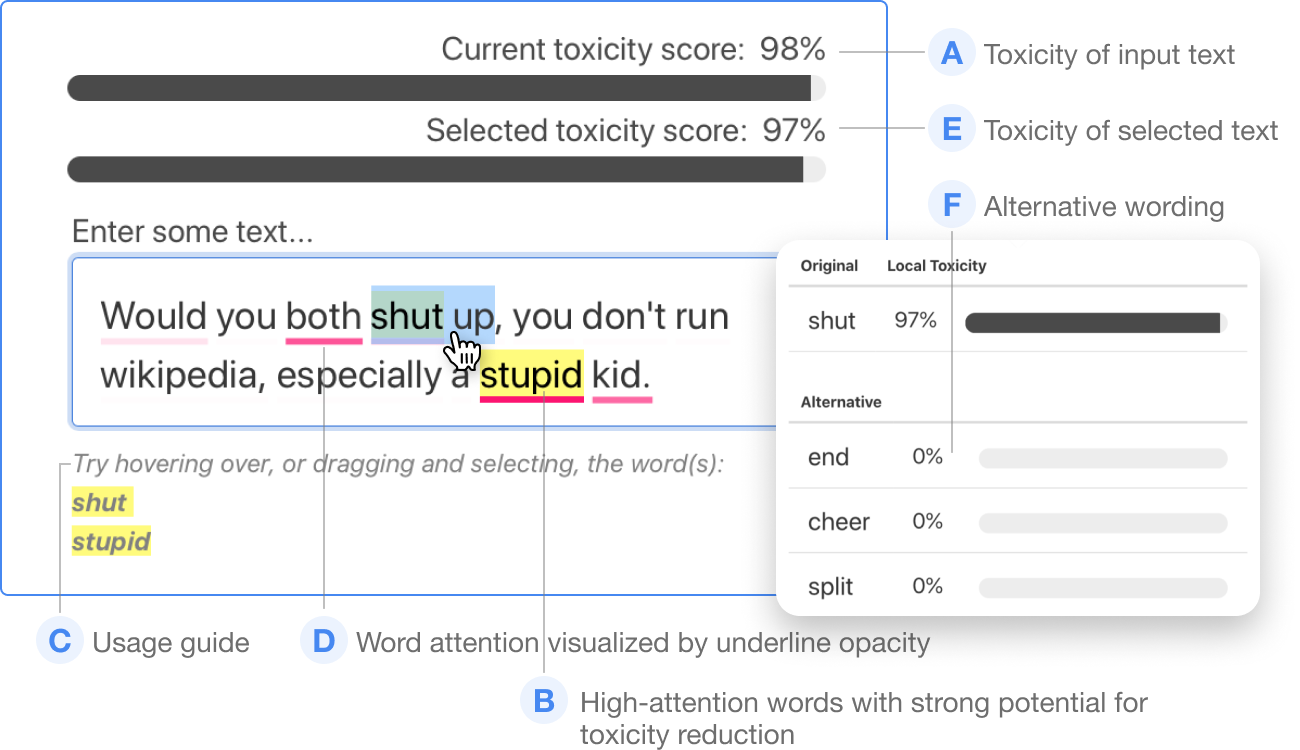
Abstract
With the widespread use of toxic language online, platforms are increasingly using automated systems that leverage advances in natural language processing to automatically flag and remove toxic comments. However, most automated systems, when detecting and moderating toxic language, do not provide feedback to their users, let alone provide an avenue of recourse for these users to make actionable changes. We present our work, RECAST, an interactive, open-sourced web tool for visualizing these models’ toxic predictions, while providing alternative suggestions for flagged toxic language. Our work also provides users with a new path of recourse when using these automated moderation tools. RECAST highlights text responsible for classifying toxicity, and allows users to interactively substitute potentially toxic phrases with neutral alternatives. We examined the effect of RECAST via two large-scale user evaluations, and found that RECAST was highly effective at helping users reduce toxicity as detected through the model. Users also gained a stronger understanding of the underlying toxicity criterion used by black-box models, enabling transparency and recourse. In addition, we found that when users focus on optimizing language for these models instead of their own judgement (which is the implied incentive and goal of deploying automated models), these models cease to be effective classifiers of toxicity compared to human annotations. This opens a discussion for how toxicity detection models work and should work, and their effect on the future of online discourse.
Materials
BibTeX
@article{10.1145/3449280,
author = {Wright, Austin P. and Shaikh, Omar and Park, Haekyu and Epperson, Will and Ahmed, Muhammed and Pinel, Stephane and Chau, Duen Horng (Polo) and Yang, Diyi},
title = {RECAST: Enabling User Recourse and Interpretability of Toxicity Detection Models with Interactive Visualization},
year = {2021},
issue_date = {April 2021},
publisher = {Association for Computing Machinery},
address = {New York, NY, USA},
volume = {5},
number = {CSCW1},
url = {https://doi.org/10.1145/3449280},
doi = {10.1145/3449280},
journal = {Proc. ACM Hum.-Comput. Interact.},
month = apr,
articleno = {181},
numpages = {26},
keywords = {natural language processing, interactive visualization, toxicity detection, intervention, content moderation}
}